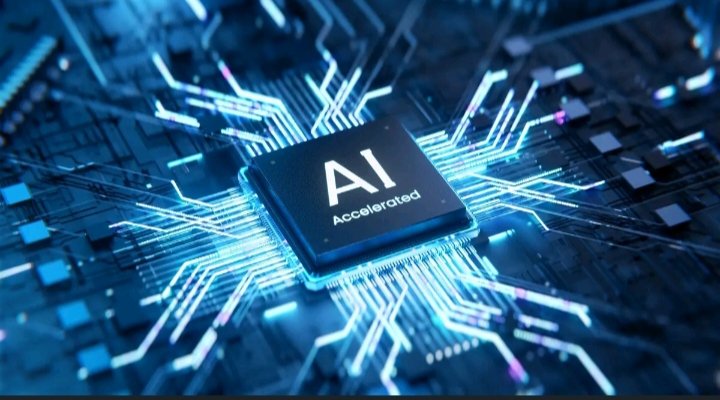
The Evolution of AI Chips: Powering the Future of Artificial Intelligence
Introduction
Artificial Intelligence (AI) has rapidly evolved from a theoretical concept to a transformative force across various industries. At the heart of this revolution are AI chips, specialized processors designed to accelerate AI tasks such as machine learning, computer vision, and natural language processing. This article explores the evolution, types, and significance of AI chips in shaping the future of technology.
Understanding AI Chips
AI chips, also known as AI accelerators, are semiconductor devices optimized for AI workloads. Unlike traditional CPUs and GPUs, which are more generalized in their computing capabilities, AI chips are designed specifically to perform the complex mathematical calculations essential for AI applications with high efficiency and speed.
Types of AI Chips
AI chips can be broadly categorized into two types based on their architecture and function:
- CPU-Based AI Chips
- These chips integrate AI capabilities into traditional central processing units (CPUs).
- They are versatile and widely used in general-purpose computing tasks.
- Examples include Intel Xeon processors with integrated AI features.
- GPU-Based AI Chips
- Graphics Processing Units (GPUs) adapted for AI tasks.
- Known for their parallel processing power, making them ideal for deep learning algorithms.
- NVIDIA Tesla GPUs are prominent examples used extensively in AI research and applications.
- ASIC-Based AI Chips
- Application-Specific Integrated Circuits (ASICs) optimized for AI workloads.
- Designed for maximum performance in specific AI tasks, such as inferencing or training.
- Google’s Tensor Processing Units (TPUs) exemplify ASICs tailored for machine learning tasks.
Each type of AI chip offers unique advantages in terms of speed, efficiency, and cost-effectiveness depending on the specific requirements of AI applications.
Evolution of AI Chips
The evolution of AI chips has been marked by significant advancements in performance and efficiency, driven by the increasing demand for AI capabilities in diverse fields such as healthcare, finance, automotive, and more.
Moore’s Law and Beyond
Moore’s Law, which states that the number of transistors on a microchip doubles approximately every two years, has influenced the continuous miniaturization and increased computing power of AI chips. However, as transistor sizes approach physical limits, innovators are exploring new architectures and materials to sustain performance gains.
From CPU to GPU Dominance
Initially, CPUs were the primary choice for AI tasks due to their versatility. However, the rise of deep learning algorithms necessitated more parallel processing capabilities, leading to the adoption of GPUs. GPUs accelerated the training of neural networks by distributing computations across multiple cores, significantly reducing training times.
Emergence of ASICs and TPUs
the limitations of GPUs in terms of power consumption and efficiency spurred the development of ASICs tailored specifically for AI tasks. ASICs, such as Google’s TPUs, are designed to handle large-scale AI workloads more efficiently than traditional processors, making them ideal for applications requiring real-time data processing and low-latency responses.
Applications of AI Chips
AI chips are integral to a wide range of applications that leverage AI technologies to enhance efficiency, accuracy, and automation across industries. Some notable applications include:
- Autonomous Vehicles
- AI chips enable real-time processing of sensor data for navigation, object detection, and decision-making in autonomous vehicles.
- Healthcare
- AI chips power medical imaging systems for faster and more accurate diagnostics, personalized treatment recommendations, and drug discovery.
- Finance
- In the financial sector, AI chips facilitate algorithmic trading, fraud detection, and risk assessment with high-speed data analysis.
- Smartphones and Consumer Electronics
- Mobile devices equipped with AI chips support features like voice recognition, image enhancement, and augmented reality applications.
Performance Metrics and Benchmarks
AI chips Assessing the performance of AI chips involves several key metrics that highlight their capabilities in various AI tasks. Common benchmarks include:
- TOPS (Trillions of Operations Per Second): Measures the number of operations a chip can perform in a second, indicating processing speed.
- Power Efficiency: Indicates how efficiently a chip uses energy to perform computations, crucial for applications requiring low power consumption.
- Accuracy and Precision: Evaluates the accuracy and precision of AI models accelerated by the chip, impacting the reliability of AI-driven decisions.
Table 1: Comparison of AI Chip Performance Metrics
AI Chip | TOPS | Power Efficiency (TOPS/Watt) | Application Example |
---|---|---|---|
Intel CPU | 100 | 1.5 | General computing tasks |
NVIDIA GPU | 500 | 3.0 | Deep learning algorithms |
Google TPU | 1000 | 15 | Neural network inference |
Future Trends and Challenges
AI chips Looking ahead, the field of AI chips is poised for further innovation and expansion. Future trends include:
- Edge Computing: AI chips optimized for edge devices to process data locally, reducing latency and bandwidth requirements.
- Hybrid Architectures: Combining different types of AI chips (e.g., CPUs, GPUs, ASICs) for enhanced performance and flexibility in AI applications.
- Ethical Considerations: Addressing ethical implications such as data privacy, bias in AI algorithms, and environmental sustainability in chip manufacturing.
Conclusion
AI chips represent a pivotal advancement in semiconductor technology, driving the widespread adoption and innovation of AI across industries. As demand for AI capabilities continues to grow, the evolution of AI chips will play a crucial role in shaping the future landscape of technology, enabling smarter, more efficient, and more capable AI systems.
In summary, AI chips are not just components but enablers of a transformative era where intelligent machines enhance human capabilities and drive innovation at an unprecedented pace.
By exploring the evolution, types, applications, and future trends of AI chips, this article aims to provide a comprehensive understanding of their significance in advancing AI technologies and their impact on society.